Data integrity is a foundational element of any successful machine learning project. Inaccurate or inconsistent data can lead to flawed models and unreliable results. In this blog, we’ll discuss the importance of data integrity in data science and share best practices for ensuring your data is clean, accurate, and ready for machine learning.
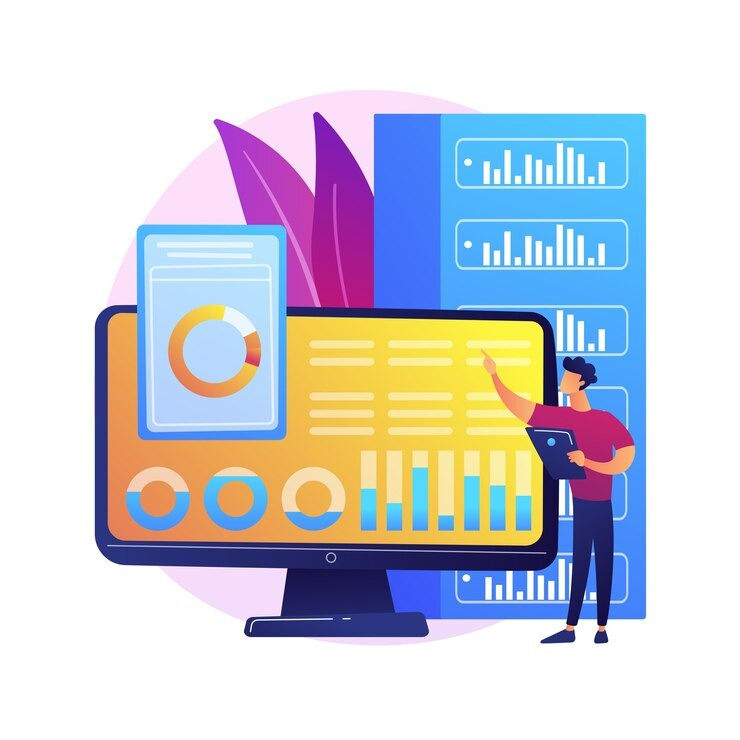
What is Data Integrity?
Data integrity refers to the accuracy, consistency, and reliability of data throughout its lifecycle. In machine learning, data integrity ensures that the data used for training models is accurate, complete, and free from errors.
The Importance of Data Integrity in Machine Learning
Machine learning models rely on high-quality data to make predictions. If the data is flawed or incomplete, the model’s predictions will be inaccurate, which can lead to poor decision-making and business outcomes. Ensuring data integrity is critical for:
Improving Model Accuracy: Clean, accurate data allows machine learning algorithms to learn better and produce more reliable results.
Ensuring Ethical Decision-Making: Data integrity helps avoid biased or discriminatory outcomes, which can occur when training data is incomplete or skewed.
Best Practices for Ensuring Data Integrity
Data Validation: Regularly check data for consistency, completeness, and accuracy before feeding it into machine learning models.
Error Detection and Correction: Use automated tools to identify and correct data errors, such as duplicates or outliers.
Data Preprocessing: Proper preprocessing steps, including normalization and standardization, can help ensure that the data is suitable for model training.
Data Audits: Conduct routine audits to ensure that your data remains accurate and relevant throughout the lifecycle of the machine learning project.
Conclusion
Data integrity is essential for building reliable machine learning models. By following best practices for ensuring clean, accurate data, data scientists can build models that deliver trustworthy results and drive better business decisions.
Comentários