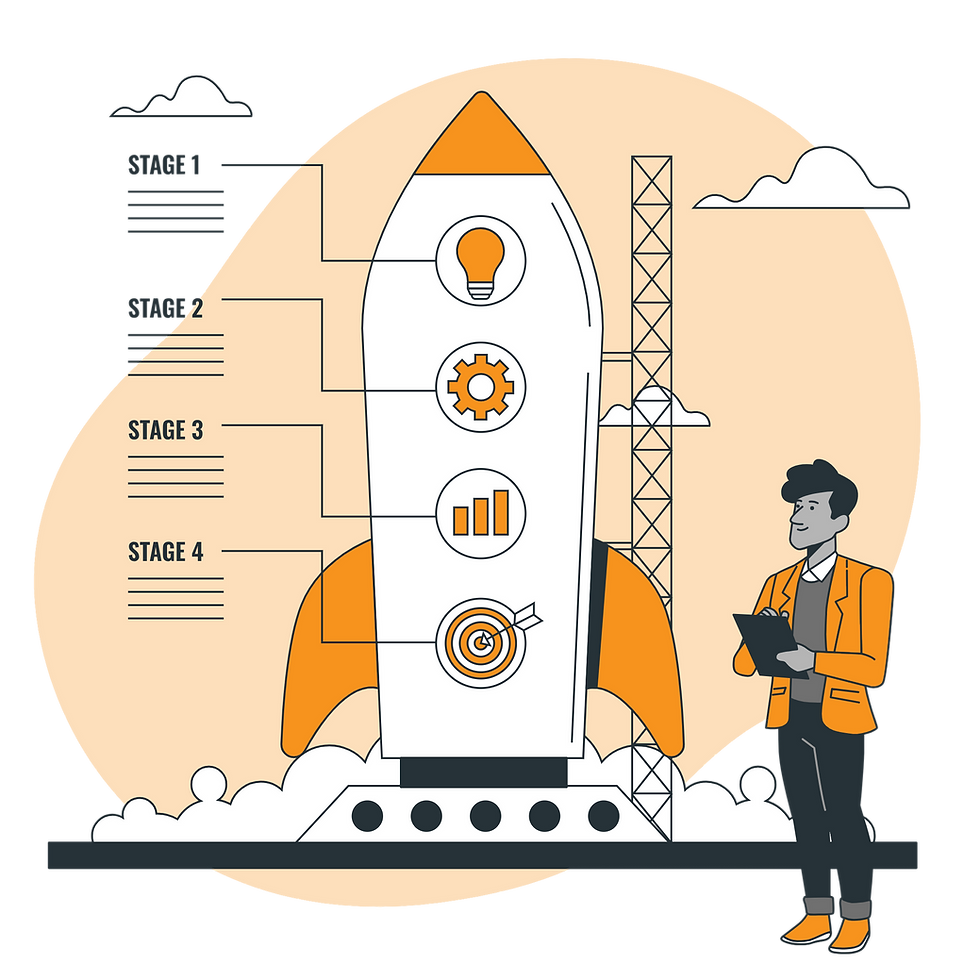
Data scientists are the modern-day alchemists, transforming raw data into insights that drive business growth. But just like any alchemist needs a clear recipe for success, data scientists need well-defined Key Performance Indicators (KPIs) to measure their impact and navigate the complex world of data analysis. So, how do you craft KPIs that unlock the full potential of your data science team?
Here is a step-by-step process for defining KPIs:
Step 1: Align with Business Goals
Your first step is to escape the data silo. KPIs for data scientists shouldn't exist in isolation. They must be firmly anchored in the broader business objectives. Collaborate with stakeholders to understand the organization's strategic priorities and identify areas where data analysis can make a tangible difference. Is it boosting revenue, optimizing customer experience, or mitigating risk? Aligning your KPIs with these overarching goals ensures your data scientists are contributing directly to the organization's bottom line.
Step 2: Break it Down
Once you have the big picture, zoom in on specific projects or initiatives. Each project should have its own set of KPIs, tailored to its unique goals. For example, an anomaly detection project might focus on reducing false positives, while a customer segmentation project might aim to increase conversion rates within a specific segment. By defining distinct KPIs for each project, you create a clear roadmap for success and hold your data scientists accountable for specific outcomes.
Step 3: Embrace SMARTness
Remember, good KPIs are SMART: Specific, Measurable, Achievable, Relevant, and Time-bound. Don't fall into the trap of vague metrics like "improved model accuracy." Instead, define concrete measures like "increase model accuracy to 95% for churn prediction within Q3." This level of specificity makes it clear what success looks like, how progress will be tracked, and whether the goals are realistic within the given timeframe.
Step 4: Think Beyond Models
While model performance is a crucial element, don't limit your KPIs to technical metrics. Remember, data science is about driving business impact. Consider KPIs that measure the real-world outcomes of your projects. For example, track how a pricing optimization model translates to increased revenue, or how a customer churn prediction model impacts retention rates. This holistic approach ensures your KPIs capture the full value of your data science efforts.
Step 5: Embrace Iteration
The data science landscape is constantly evolving, and so should your KPIs. Regularly review and refine your metrics to ensure they remain relevant and aligned with changing business priorities. Don't be afraid to adjust targets or even scrap KPIs altogether if they no longer serve a purpose. This iterative approach ensures your KPIs stay dynamic and continue to guide your data scientists toward impactful and successful outcomes.
Bonus Tip: Foster a culture of collaboration and transparency. Involve your data scientists in the KPI definition process. Their expertise and insights can be invaluable in crafting metrics that are both meaningful and achievable.
By following these steps, you can move beyond the realm of guesswork and equip your data science team with the clear direction they need to navigate the complex world of data analysis. Remember, well-defined KPIs are not just measuring sticks; they are powerful tools that unlock the true potential of your data science team and propel your organization toward data-driven success.
So, chart your course, define your KPIs, and embark on a journey of data-driven transformation with your data science team as your skilled navigators. The future of your organization lies hidden within the data, and with the right KPIs, you can unlock its immense potential.
I hope this blog provides a valuable roadmap for defining KPIs for your data scientists. Feel free to share your insights and experiences in the comments below! Let's navigate the data-driven future together!
Comments